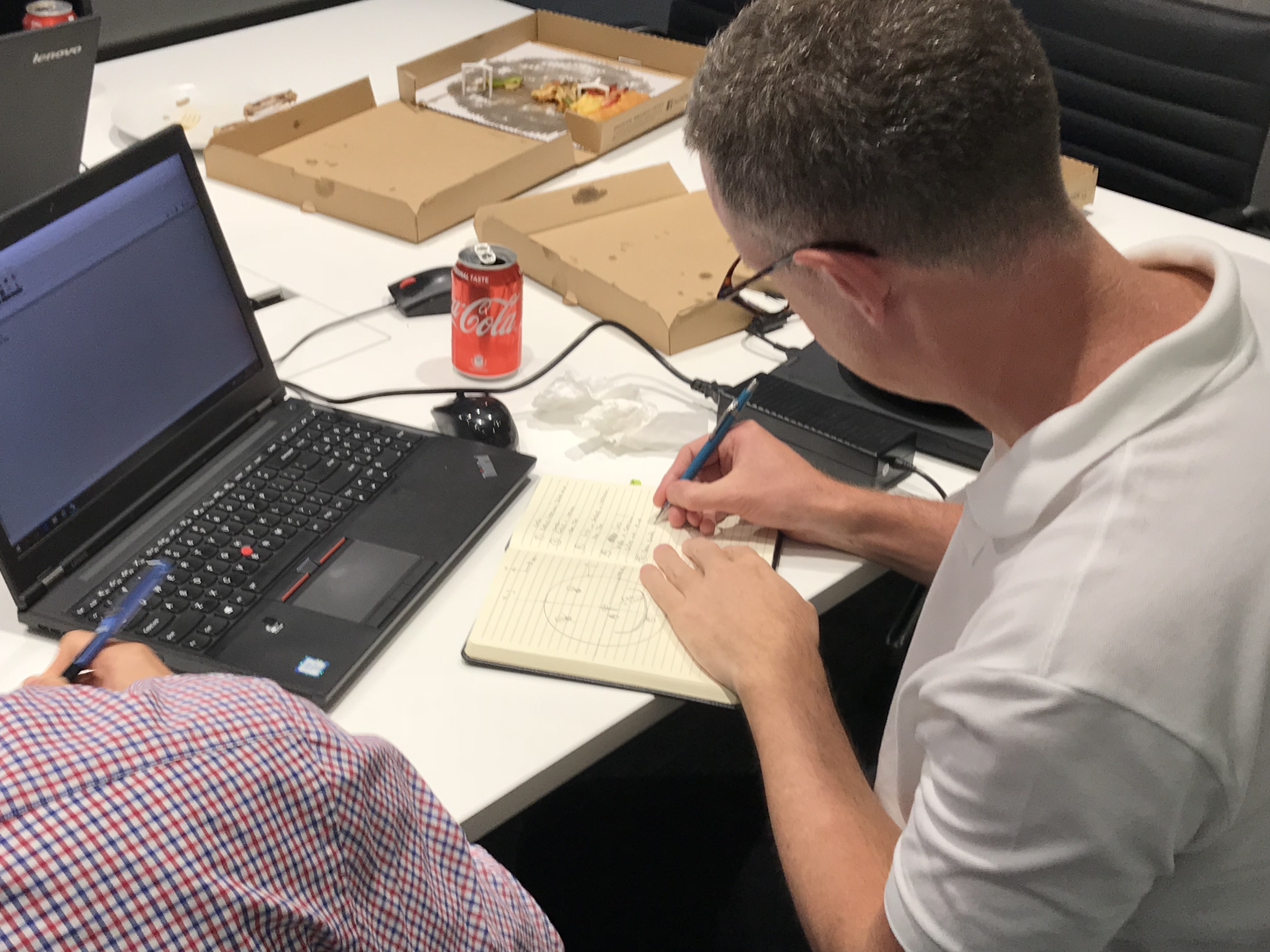
3 Lessons Learnt after 1 Year of Training an Engineering Office in Computation
Transforming an Engineering Office
One year ago this week, we commenced our lunchtime Computational training sessions at BuroHappold’s Hong Kong office. These lessons are one of the three main pillars of our Computational Transformation in Asia, which I am leading. The goal – mass participation in computation where all engineers in the office utilize code as part of their daily work. Throughout the year, I’ve run an average of 3 lunch sessions per week focused on learning Computational first-principles which will be useful to any engineer looking to augment their expertise with computation. Formulating and leading these sessions has been an amazing and fulfilling experience. Not only have I been fortunate to learn about the specific digital issues of many disciplines from my talented colleagues, but also, I’ve learned a few key things about what’s holding the AEC industry back from mass adoption of computation. Here’s my three key take-aways from 1 year and 150 lunchtime sessions of training an engineering office in computation.
The Scale of Learning and Implementing Computation for an AEC Business
The biggest lesson I’ve learned is the scale of the commitment for our industry to transform digitally, modernized, and adopt computation. The scale of the commitment is something some of us who are already adept in using computation on projects take for granted. After many years of executing jobs computationally, shouting about the immense power of computation methods, and running hack sessions to encourage others, I still didn’t see others picking it up. “Why are more people not doing this stuff?” I would often wonder. I had lost touch with how many hours I’d actually spent since 2011 hacking away at school, work, late at night, and over weekends to become fluent. I was grossly underestimating the scale of what it takes to become a Computational Engineer for the average practicing engineer. This was one of my goals for these sessions – to gain empathy for the scale of becoming a commercially viable Computational Engineer and find out how many hours it takes to become fluent in Computational Engineering skills.
After a year training an engineering office in computation, I can confidently tell you the scale of learning computation is equivalent to a full time commitment for months in a row. Even 1 lunchtime session per week for a year, surrounded by folks who can help, and a pre-engineered curriculum doesn’t suffice. Don’t get me wrong – those sessions will be useful and you will gain skills. Yet, the learning is only one facet of the lessons. The critical challenge is for these sessions need to have a payoff for the business. The problem is even after a year’s worth of once-a-week lessons, you’ll still just be scratching the surface of what you need to know to be commercially viable. Commercially viable meaning you are fluent and confident enough to do it computationally, knowing that in the short term, you will fall behind the guys doing it manually, but soon, you will zoom past them:
The AEC industry must be empathetic to the scale of this computational transition we must make if we are to shake our reputation as an industry stuck in the past. We are no longer talking about adopting easy to use, off-the-shelf software as we have in the past – this stuff takes a bigger commitment. A lunchtime session once a week, whilst helpful, should be considered a bare minimum investment. The scale of the commitment begs that we mix computation into our business as usual and the expectation that the payoff from investment will not yield instant results. Initially you will be less productive using computation. This means changing more than just what software we use, but rather, how we approach our projects.
Smart People are Already Biased (or Confused)
Being a practicing structural engineer, I’m fairly familiar with the issues related to structural engineers adopting computation. As part of these training sessions, I was exposed to the biases of Mechanical, Electrical, Plumbing, Facade, and Building Physics Engineers. One of the best parts of teaching many different disciplines was seeing how other disciplines interpret what it means to be digitally fluent. No matter how brilliant you are as an engineer, you probably have biases based on software – something Mario Carpo warned us about:
“All tools feed back onto the actions of their users, and digital tools are no exception. All design software tends to favor some solutions to the detriment of others…”
-Mario Carpo, “The Alphabet and the Algorithm”
I’ve noticed Structural and Facade engineers tend to be more geometrically inclined than MEP engineers. As such, there’s a bias of Structural and Facade engineers towards Grasshopper and MEP engineers towards Dynamo. Once someone has it in their head that “Software XYZ” or “Programming Language XYZ” is for another discipline, they promptly write off those tools along any potential benefits those tools may have in store for them. What’s more, the biases incurred from years of use of standard software has taken their toll on our mindsets. Some are still confused as to why we need computation – BIM is the be all and end all of tech in the AEC, right?
I’ll give you an example. Here is data from a Revit model which I had scraped and visualized with Grasshopper using BHoM:
In the video, we portray tree hierarchy in Revit spatially with a series of arrows. When my Electrical colleagues saw this, many said “Wow, I would have never thought to do that”. This to me is their biases towards BIM and Dynamo coming home to roost. Could you generate this diagram in Dynamo? Yes. Is it easier, more convenient and flexible in Grasshopper? I would (as someone who uses both) say yes. Grasshopper is a great medium for visualizing data in some ways but also, a bit less clunky than Dynamo for these kind of things. It also presents advantages because you can “bake” your data and use Rhino as your “chalkboard” to manually investigate and understand the data. But hey, I’m a structures guy, so maybe I’m biased! The key here isn’t an argument over what software is best, but rather, the statement that “I would have never thought of that” was due to inherent bias in someone’s thinking.
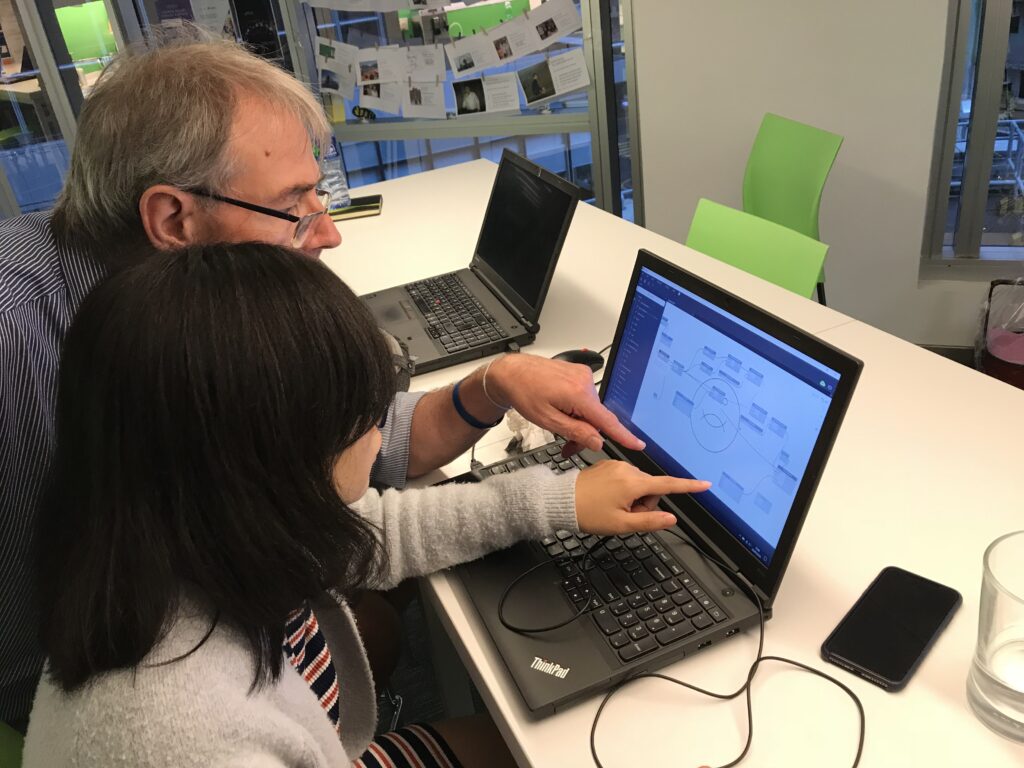
We could all do with a bit of working together to expose these biases and start thinking in new ways. Biases will instantly cause good engineers to miss out on easy computational wins. To break these biases and get everyone to understand that perfect tools do not exist has been a key challenge to solve over as part of these sessions.
Everybody Learns in Different Ways
Our Hong Kong office is one of the most diverse office you will ever find – we have folks from all over the world. I spend most my days overhearing two or three languages other than English. I hear at least that many different versions of English as well. With such a diverse set of individuals, I was a fool to think I could come up with one curriculum that would work for everyone. But alas, that is how I began. I tried to distill my 7 years worth of computational experience into a simple course. I came up with an initial curriculum which tried to be discipline agnostic, whilst remaining relevant to every discipline. The course revolved a simple idea – 🙂
Throughout the year, the curriculum for training an engineering office in computation changed as I learned from each training session the different ways engineers respond to the training. One of the main ways that it changed was the pace and complexity of each exercise. I realized quite quickly that you cannot ask much of a beginner. Equally, I found that the density of teachers to students was a key factor. The golden ratio was 1 teacher to a maximum of 3 students. If we broke that ratio, it slowed the learning curve drastically for everyone and the training was ineffective. Most likely, at the end of their first lunchtime session, students will have laid only one or two components on the Grasshopper or Dynamo canvas (again, think scale) even with the help on a expert. So the exercises had to be very simple with only one small baby step of a twist added from one exercise to the next.
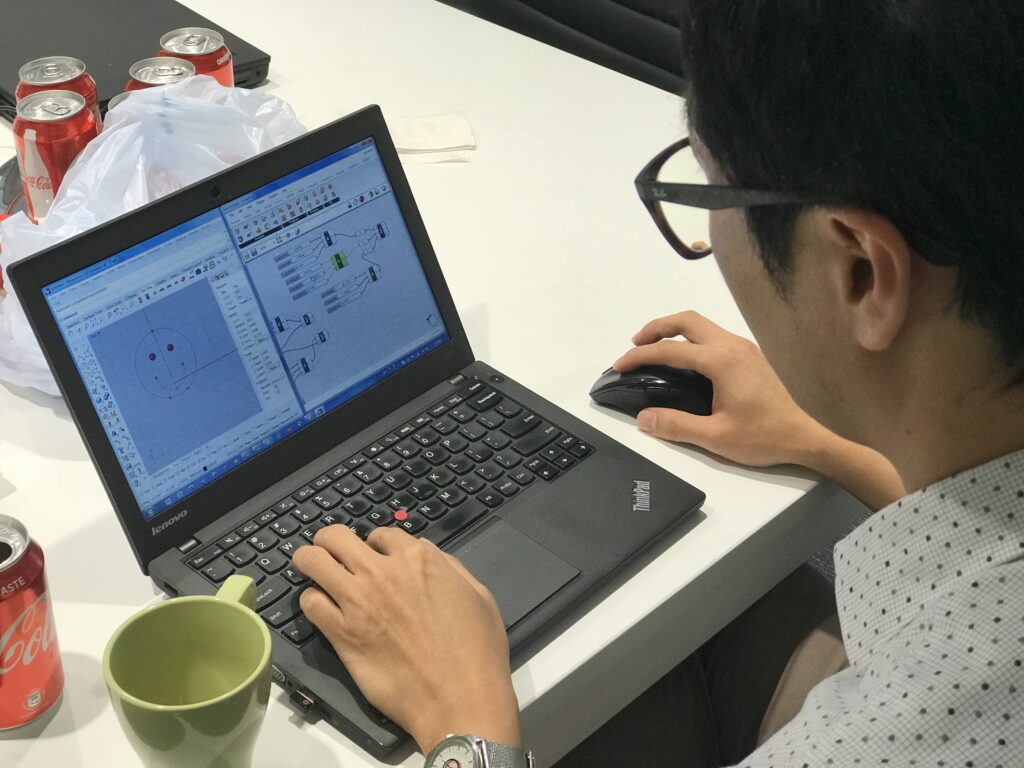
Some people really understood the agnostic principles of the ‘smiley face’ training. However, many found that it was too abstract and didn’t understand how it related to their daily work. Some needed discipline-specific, simple examples. Some needed examples related to their current project. Some needed a combination of all of these methods. Some just needed it explained in their native tongue instead of my American English. In the end, this is a challenge for an industry like AEC with a wide array of disciplines. We all think and learn differently, which makes it difficult for businesses to find a ‘one size fits all’ resource to upskill their staff in computation.
Transforming Business as Usual in AEC
So as you can imagine, the past year has been full of challenges and learning training an engineering office in computation. We’ve made quite a bit of progress, but there’s still a long way to go. However, as with all things digital, the law of exponential returns is on our side! I’m excited for the next chapter of our computational transformation and to use the lessons from last year to our advantage.
If you want to learn more about Computational Engineering, make sure to check out my Computational Engineering 101 series.